Research Interests |
 |
Living systems are made up of many different types of molecular entities, and accordingly there are different types of networks.
One of the most important types of networks exists at the layer of "gene regulation", which controls what set of genes get
expressed at any point in time and space. Our work has focussed primarily on understanding the transcriptional layer of gene regulation.
Here are some research directions that we are pursuing or wish to pursue:
|
Inference of structure and function of regulatory networks |
Regulatory networks are directed networks of genes and regulatory proteins called transcription factors.
The transcription factors are the ``controllers'' of gene expression of a gene specifying when and where a gene is expressed.
There are two parts to a regulatory network: the structure, specifying which transcription factors regulate which genes,
and the function, specifying the logic and control of how the expression level of transcription factors determine the expression of downstream genes.
Inference of a regulatory network requires that we solve both problems, i.e. find what the edges are and find what mathematical functions
precisely capture the relationship between transcription factors and output gene expression. |
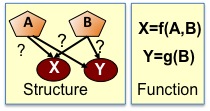 |
|
Comparative analysis of expression modules across species |
|
 |
Expression modules are sets of genes that have similar expression profiles under a given condition, often because the genes
are regulated by the same set of regulatory factors. While identifying such modules in a single species is a well-understood
problem, doing so across multiple species is still in its infancy. However, this is important, because changes in gene expression
are likely to play a major role in the evolution of complex behavior of organisms. We are interested in developing algorithms
to identify such modules across species. |
|
Evolution of gene regulation |
Identification of evolutionary principles involved in shaping and constraining regulatory and protein interaction networks. This entails learning networks across multiple species. |
Relational learning to predict function |
Modeling condition-specific functional behavior |
Learning networks of condition-specific responses, where a condition represents a global variable for a biological system (cell, tissue, organism) and affects the behavior of the system as a whole. |
Learning causal networks |
Learning causal networks describing tissue and disease-specificity. |
Learning high-resolution networks, that is networks where edges represent physical interactions, rather than functional
interactions among nodes. Such networks include entities of many different types, some of which we cannot directly (protein and metabolite
expression) requiring hidden variable inference algorithms. |
Predictive models of phenotypic response |
|